Search here
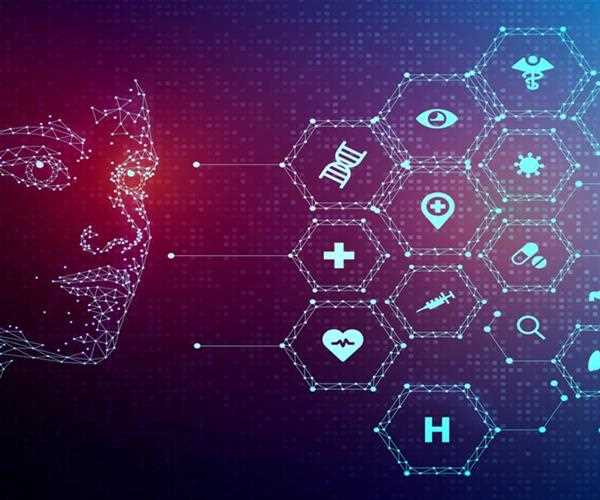
10-Jan-2023
Predictions of human illness with AI and machine learning
Playing text to speech
Many of us have heard about the advances being made in artificial intelligence (AI) and machine learning, but few are aware of the true potential these technologies have in healthcare. AI and machine learning can be used to accurately predict illnesses and diseases before they occur, enabling early detection and potentially saving lives. In this blog post, we will explore how AI and machine learning are being used to predict human illness, as well as some of the ethical considerations that come with such powerful technology. We will also look at how predictive analytics can be used to ensure better patient care in the future.
What is AI and machine learning
- AI is a broader concept that refers to machines that can carry out tasks that would normally require human intelligence, such as understanding natural language and recognizing objects. Machine learning, on the other hand, is a subfield of AI that deals with giving computers the ability to learn from data without being explicitly programmed.
- Machine learning algorithms have been able to accomplish some impressive feats in recent years, such as beat humans at complex games like Go and poker, but they are still far from perfect. One area where machine learning is beginning to show a lot of promise is in the field of medicine. Researchers are using machine learning algorithms to analyze medical data in order to make predictions about human health.
- One recent study used machine learning to predict which patients were at risk of developing dementia. The algorithm was able to accurately predict which patients would develop dementia within five years with an accuracy of 82%. Another study used machine learning to predict heart attacks with an accuracy of 90%.
- These studies show that machine learning can be a powerful tool for predicting human illness. As more data is collected, it is likely that machine learning algorithms will become even more accurate in their predictions.
How can AI and machine learning be used to predict human illness
- AI and machine learning can be used to predict human illness by analyzing patterns in a person’s medical history, symptoms, and lifestyle. This information can be used to create a model that can identify risk factors for certain diseases and make predictions about a person’s likelihood of developing them.
- Machine learning is particularly well-suited to this task because it is able to learn from data sets that are too large or complex for humans to analyze directly. By using machine learning, we can develop models that can detect subtle patterns that might otherwise be missed.
- In recent years, there have been exciting advances in the use of machine learning to predict human illness. For example, Google’s DeepMind Health team has developed an algorithm that can predict the onset of acute kidney injury up to 48 hours in advance with 90% accuracy. This type of technology has the potential to transform healthcare by allowing us to intervene early and prevent potentially life-threatening illnesses.
What are the benefits of using AI and machine learning to predict human illness
- There are many potential benefits to using AI and machine learning to predict human illness.
- First, AI and machine learning can help us to identify patterns in data that we may not be able to see with the naked eye. This can lead to earlier detection of illness, which can be crucial in preventing further health complications.
- Second, AI and machine learning can help us to understand the root causes of illness. This knowledge can then be used to develop targeted treatments and prevention strategies.
- Third, AI and machine learning can help us to personalize medicine. By understanding an individual’s unique physiology, we can develop treatments that are much more likely to be effective for that person.
What are the limitations of using AI and machine learning to predict human illness
- There are a number of limitations to using AI and machine learning to predict human illness.
- First, these technologies are still in their infancy and have a lot of room for improvement.
- Second, they rely on large data sets to be effective, which can be difficult to come by.
- Third, they are not always accurate, and even when they are, there is always the potential for error.
- Fourth, they can be expensive to implement and maintain. Finally, they may not be able to take into account all of the factors that can influence a person’s health.
How accurate are predictions made by AI and machine learning
- There is no simple answer to how accurate predictions made by AI and machine learning are. In general, these methods are good at making predictions based on data, but they can be limited by the quality of the data. For example, if there is a lot of missing data, or if the data is not representative of the population as a whole, then the predictions may not be accurate.
- AI and machine learning algorithms can also be biased if the training data is not diverse enough. For instance, if the data only contains information about white males, then the algorithm may have difficulty accurately predicting for other groups of people.
Conclusion
AI and machine learning are transforming the field of health care, providing us with better tools to predict illnesses in humans. With the help of these technologies, we can now identify potential diseases earlier than ever before – sometimes even before symptoms appear. This could mean a huge breakthrough in preventative medicine, saving millions of lives in the years to come. While there is much work to be done when it comes to perfecting AI-driven medical diagnostics, its potential is clear and promises an exciting future for healthcare as we know it today.
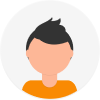
Comments
Solutions
Copyright 2010 - 2024 MindStick Software Pvt. Ltd. All Rights Reserved Privacy Policy | Terms & Conditions | Cookie Policy