Search here
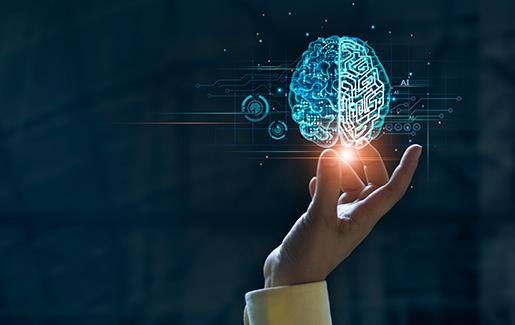
data science
05-Sep-2022
How machine learning helps us to understand the brain
Playing text to speech
Machine learning can help us understand the brain in a variety of ways. For example, it can help us learn the best way to make decisions using the information that is in our brains. This research has led to advances in Artificial Intelligence and computer vision. Ultimately, this knowledge can help us create better products for humans.
Reinforcement learning
- Reinforcement learning describes how our brain learns to act in ways that will produce desirable outcomes. Reinforcement learning works by modeling the decision-maker as a control system and developing strategies for making the environment behave in ways that favor the decision-maker. A simple strategy involves mapping a situation to a probability distribution, and the decision-maker chooses an action based on that probability distribution. As the decision-maker learns more about its environment, the strategy may be changed.
- Reinforcement learning is applied to a variety of AI problems. A good example is a game. A machine that uses reinforcement learning would learn to find the best action in a given situation. For example, if it were given a set of options and was rewarded for choosing a particular action, the agent would select the one that would bring the highest rewards. If the decision was the wrong one, the machine would avoid the decision and seek another one that would yield a higher reward.
- Reinforcement learning involves multiple neural networks. A single agent may know everything about the environment, but it may still face a reinforcement-learning problem that is difficult to solve. For example, a puzzle solver might know how to solve the Rubik's cube, but still experience difficulty. Nevertheless, the agent's ability to control the environment is limited.
Manifold learning
- Machine learning has the potential to help us understand the brain. It can help us manage large amounts of data and find connections that we may not have seen otherwise. It has also been used to study brain diseases. Scientists at Waisman Center are using this technology to help them better understand the brain.
- For example, machine learning algorithms can aid in hypothesis testing and data processing. Researchers have used machine learning algorithms to understand hippocampal-prefrontal interactions. Using an inhibitory strategy, they found that the ventral hippocampus's projection to the medial prefrontal cortex (mPFC) is essential for spatial cue encoding. They then trained a classifier to identify the patterns of mPFC population firing rates.
- The Hebbian rule describes how neurons learn. The more similar their activity, the more synaptic connections they will form. However, this rule is not suitable for large networks of neurons. The neural networks of the brain must learn from each other, and learning through error is not sufficient.
Natural language processing
- As neuroscience research advances, new technology is helping scientists build more accurate models of brain function. AI algorithms are now being used to study the brain's activity, as well as the connections between neurons. While this technology can help scientists find more specific details of brain activity, it is far from perfect. Scientists still need to decide which model will work best for their research.
- While it's clear that machine learning algorithms are not perfect, one key benefit is that they are more flexible than traditional algorithms. For example, neural networks are flexible enough to adapt to new data. For instance, if we want to create a machine that can distinguish between cats and dogs, we need to build a flexible classifier.
- The neural networks in the brain are complex and impacted by many factors. Dr. Daifeng Wang, a professor in the Waisman Center, and his team use artificial intelligence and machine learning techniques to understand these complex networks. These techniques may even help us predict certain disorders of the brain.
Predictive coding
- Predictive coding helps us to understand how the brain processes information. The brain uses this method to create a structured body of information from a variety of sensory inputs. It explains away information that is not predicted by the brain, which reduces the amplitude of information and allows for efficient transmission. The brain then uses this information to make predictions about events and situations at a higher level, using a technique called Bayesian inference.
- Predictive coding theory proposes that the brain actively makes predictions about the future. It then matches these predictions to sensory input and takes action to minimize prediction shock. It also implicitly implies that the final processing of information occurs before perception. Top-down predictions precede the bottom-up sensory feed, which helps the brain to make predictions about future events.
- This new understanding of the brain can help us understand the connection between autism and schizophrenia. According to the theory, the brain in autism gives too much weight to sensory input, while in schizophrenia, it gives too little weight. This may explain the phenomenon of hallucinations in schizophrenia. It may also explain the emergence of delusions in autism and schizophrenia. These are different from false perceptions, but predictive coding suggests that delusions emerge when expectations are too weak.
machine learningreinforcement learningmanifold learningnatural language processingpredictive codingtop-down predictionsdecision-maker
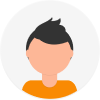
Written By
I am Drishan vig. I used to write blogs, articles, and stories in a way that entices the audience. I assure you that consistency, style, and tone must be met while writing the content. Working with th . . .
Comments
Solutions
Join Our Newsletter
Subscribe to our newsletter to receive emails about new views posts, releases and updates.
Copyright 2010 - 2025 MindStick Software Pvt. Ltd. All Rights Reserved Privacy Policy | Terms & Conditions | Cookie Policy